Assessing Chinese GenAI Applications: Why Invest and What to Consider? (2 of 2)
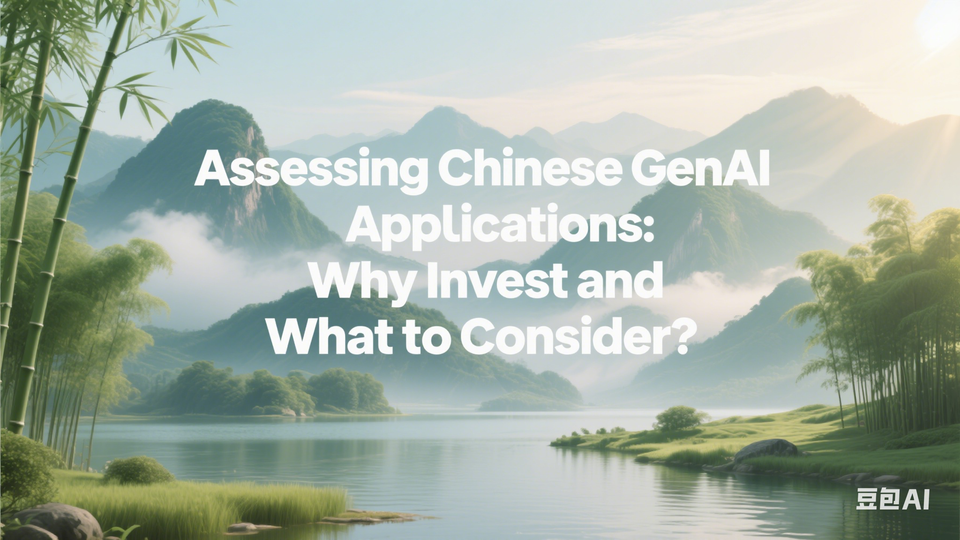
Last year, when Sand Hill Road funds went all-in on GenAI, I started to feel somewhat anxious. I was previously focused on Deep Tech investment, and I had to push myself to pivot into GenAI investing. From paying attention to a new sector to deal sourcing and researching, and then to hands-on investing, there are significant gaps to fill in. This year, after DeepSeek and Manus showing their disruptive capabilities, almost every Chinese investor started looking into AI. There was no longer any diverse opinion, and my anxiety deepened.
When meeting with founders, I found out that many of them are Gen-Z people like me, but with more first-hand knowledge about GenAI than I have. This is a whole new founder persona, different from the Deep Tech founder that I am used to co-work with. I feel easy to empathize with these founders, since I have nearly the same age as them. But I still have encountered challenges as follows:
- The industry is immense, spanning from AI infrastructure to all kinds of AI applications. From which sectors should I seek entry points?
- Are there technological barriers inherent in AI applications? If technological barriers are absent, what alternative forms of competitive advantage should AI applications develop?
- As large language models (LLMs) continue to advance in capability, will many currently popular products ultimately prove to be merely transitional in nature?
- Should I invest in AI-Native applications or AI-Powered applications?
Click here to check out the previous article.
Third question:
As large language models (LLMs) continue to advance in capability, will many currently popular products ultimately prove to be merely transitional in nature?
- I consider this from several aspects.
- First, is it possible for a single model to dominate all scenarios? Actually, this seems to be the ultimate form of AGI. But in current stage, you can observe that in an AI Agent or an Agentic Workflow, models of various parameter sizes from different suppliers are used in parallel. Not every task requires the full-scale model—some 3B models can perform certain tasks better, faster, and cheaper. Even foundation model suppliers provide models in different sizes. Different tasks require trade-offs among quality, time, and cost, making it difficult for a single model to dominate all scenarios.
- Second, is it feasible for the orchestration of several models to dominate all scenarios? Many Agent frameworks orchestrate multiple Agents—some designated for planning, others for reflection, and some for execution—to accomplish a variety of tasks. I would like to introduce a coordinate: the horizontal axis represents the degree of creativity dependency (essentially reflecting human involvement), and the vertical axis represents the degree of task standardization (whether there is a SOP or repetitive execution).Utilizing this coordinate system, tasks can be divided into four quadrants.
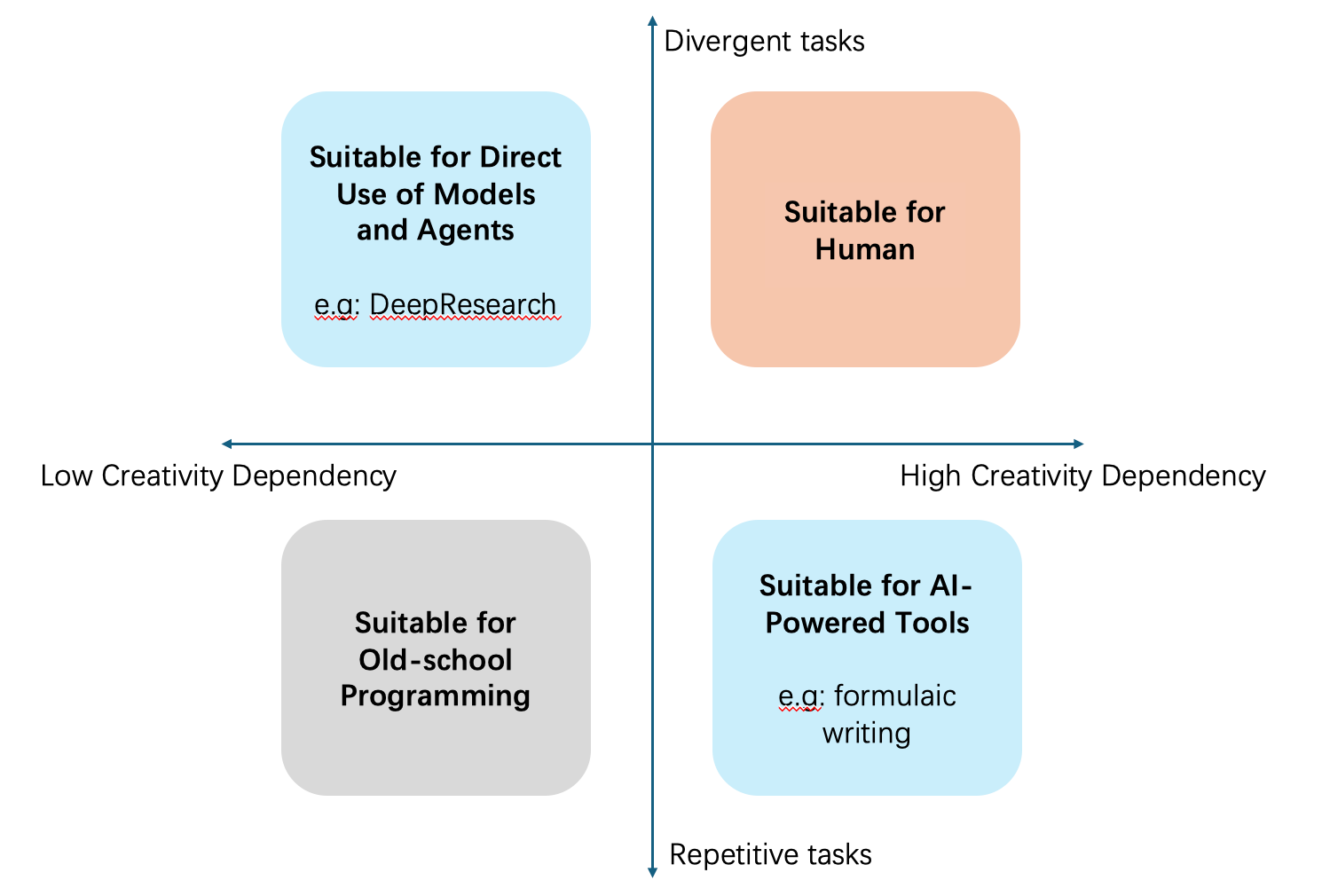
- The tasks most suitable for direct use of foundation models or general purpose agents are those with low creativity dependency and relatively divergent needs (low standardization), such as DeepResearch, where the goal is clear (researching a specific topic). Even though sometimes a universal research methodology exists, the workflow remains relatively divergent and requires flexible extraction and processing of information.
- The adjacent quadrant, characterized by low creativity dependency and highly standardized tasks, is better suited for old-school programming rather than using foundation models.
- Another quadrant, involving high creativity dependency and divergent workflows, such as art creation or cutting-edge science exploration, still presents challenges at this time and is better done by humans.
- The final quadrant, with high creativity dependency and highly standardized work, such as formulaic writing, is well-suited for AI-Powered tools.
- However, this coordinate system is not static. The quadrant suitable for the direct use of foundation models and the one suitable for AI-Powered tools are constantly encroaching on the quadrant suitable only for humans. In other words, more and more tasks traditionally suited for humans can now be replaced or partially replaced.
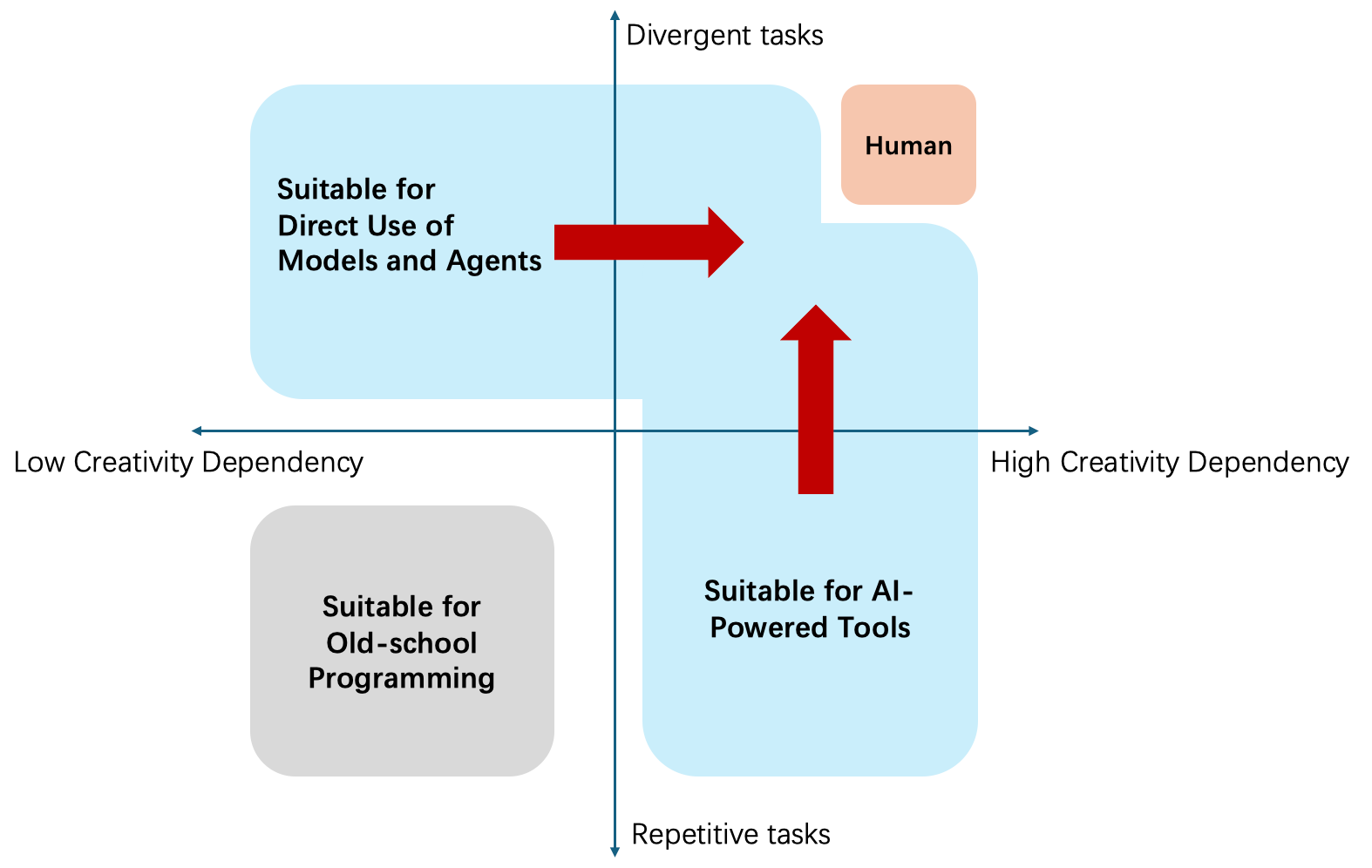
- Even within the AI-Powered tool quadrant, much of the engineering effort may be diminished by the iteration of foundation models. Eventually, it resembles a pyramid shape.
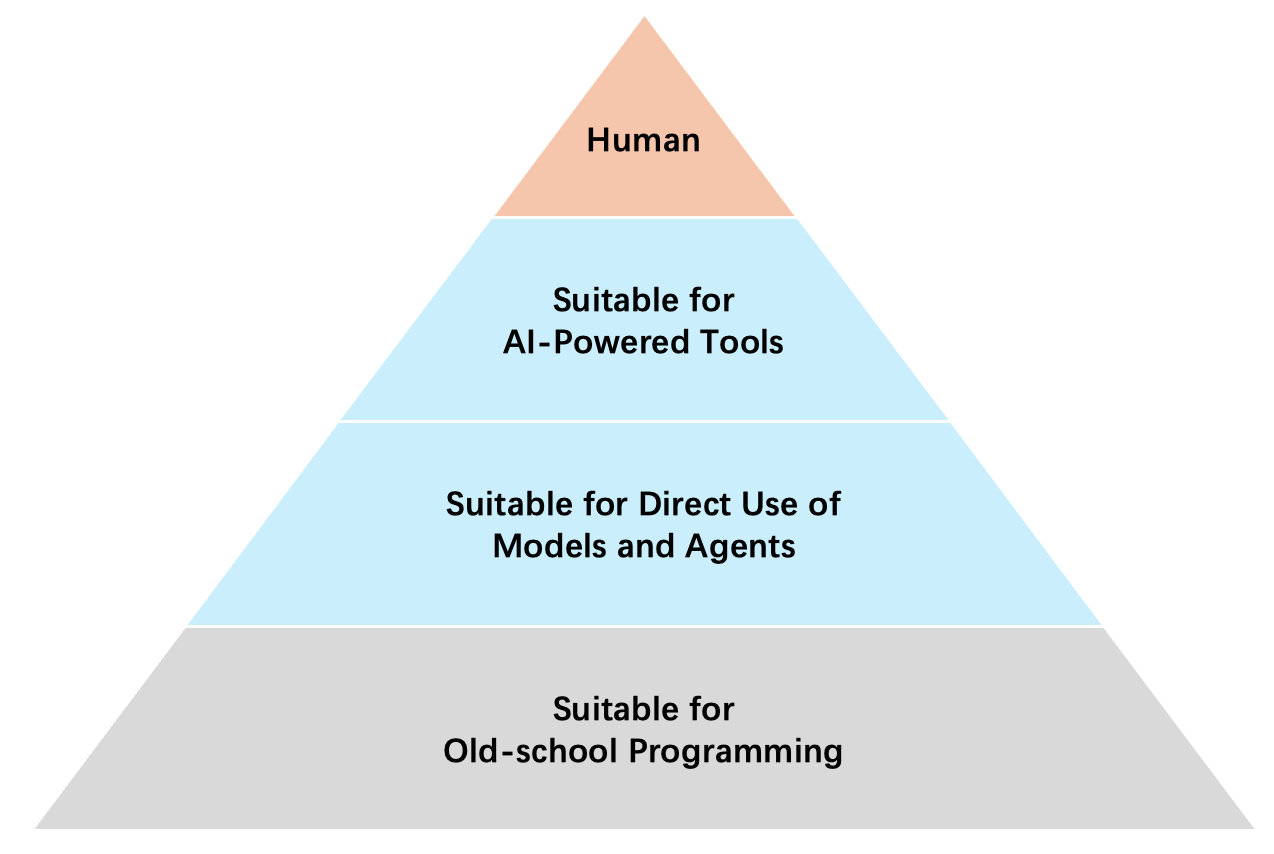
- After considering which scenarios suit foundation models/agents, AI-Powered tools, or humans, does it mean everyone should focus on developing and investing foundational models? Not necessary. Here’s my radical view: the pyramid shape above is based on workload, but if we look at value generated, it might be the opposite—a reversed pyramid shape. Deployment of model is relatively simple and quickly becomes commoditized. In contrast, the deployment of humans, as in the case of newborns, is challenging and will become increasingly scarce in the future.
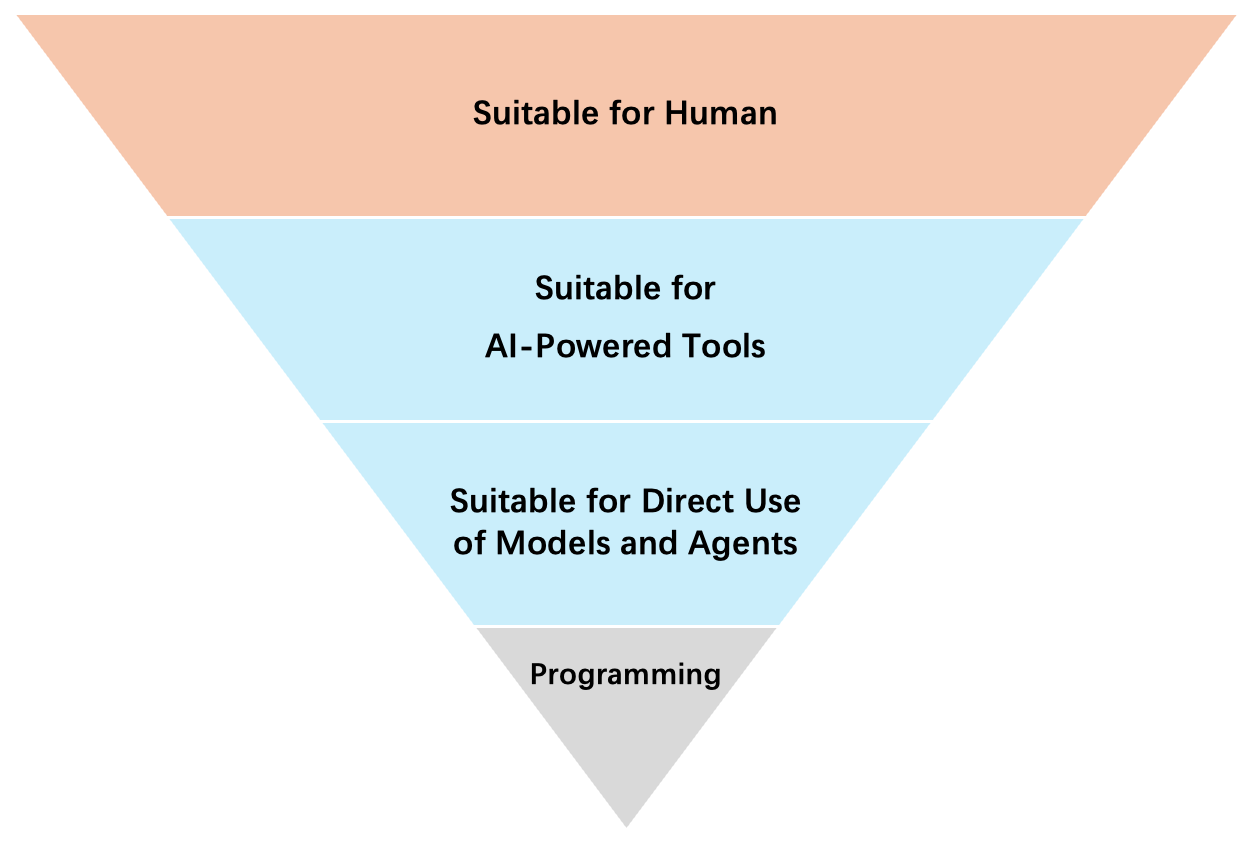
- So my radical view is to lean towards the user and humanity, where future value will be greater. In product development, one should continually move closer to the user, ultimately becoming the interface through which users understand and utilize AI. If most products are indeed transitional, this may be the key to securing an ecological niche.
This naturally leads to the fourth question:
Should I invest in AI-Native applications or AI-Powered applications?
- Firstly, AI-Native applications, or direct use of models/agents, may easily become commoditized, but that does not mean they are not valuable. Instead, they will serve as infrastructure. Foundation models will be as essential as utilities or cell networks. Token bills look like phone bills anyway. Therefore, MaaS(Model as a Service) will be the battleground for the giants.
- For startups, the goal is not to compete head-on with the giants, but to leverage resources to break through from a small entry point. This inevitably raises a key question: What is the potential size of the market?
- In deep tech investments, I have summarized two points that may also apply to AI investments:
- Existing markets are established and crowded with existing players. Startups must look for an incremental market, which is hard to estimate but has the potential to become larger. Incremental markets are vague and hard to estimate so that giants might miss them, giving startups the opportunity to deliver products first.
- Second, in hard tech, I have invested in many upstream components. These components typically have a limited market size when used in a single application, but as platform technologies, they can be applied across various applications. For example, lasers can be utilized in lithography systems, optical communication transmitters, LiDARs, and more. As platform technologies, they can "eat from multiple bowls." When investing in AI, it is crucial to consider from the outset how to expand the user base and ultimately build a platform. Whether in deep tech or AI, what eventually leads a startup to an IPO often differs from initial expectations. This requires a flexible team, with founders and investors maintaining an open mindset.